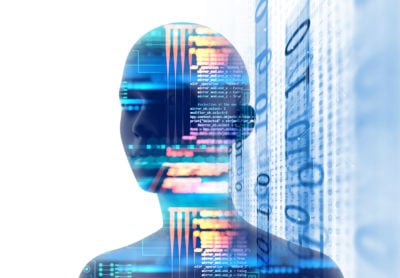
By Michael Solomon, PhD, MBA, Practice Lead, eCare Management
With billions of dollars flowing into artificial intelligence (AI) technology for healthcare, it’s no surprise that AI is rising to the peak of inflated expectations on Gartner’s hype cycle of emerging technologies. Although AI is a promising technology to tackle problems in the complex, data-intensive world of healthcare, where to place investment bets in terms of expertise, software, use cases, etc., to deliver actual value is a burning issue in the midst of all the hype.
As Point-of-Care Partners often does for clients who ask for clarity about emerging technologies, we set out to determine what specific AI technologies are real and how they are solving problems in a major area of our management consulting: the steps of medication management.
Let’s first establish what real-world AI technology exists – that is, software being deployed today to improve healthcare. Two types of AI technology are gaining real traction in health care:
- Machine learning is the most prevalent type of AI technology. Its algorithms use heuristic techniques such as mixing/matching and trial and error to improve and adapt models automatically (i.e., without programmer intervention). It has application in areas where traditional statistics start to run out of steam, such as with larger datasets and where it’s not clear what assumptions or models should be used.
- Natural Language Processing (NLP) uses a combination of expert-rules and AI techniques to process unstructured free text, such as clinical notes and transform it into structured data for more accurate searches/discovery and decision support. Advances in the technology and the explosion of computerized clinical notes are driving NLP into the mainstream of health IT.
Other emerging AI technologies (e.g., artificial neural networks, deep learning) show promise, but we’re probably a few years away from significant, real applications in healthcare – especially in the area of medication management.
Reflecting on persistent problem areas in medication use and therapy, AI has the potential to increase value in three areas in particular:
- Reduce diagnostic and therapeutic errors by evaluating large sets of data against complex, multi-factor and dynamically changing criteria (such as drug utilization review);
- Reliably and cost-effectively predict health risks and outcomes across large populations
- Reduce time and expense while increasing the reliability of repetitive analytic tasks (such as prior authorization);
These opportunities are reflected in Accenture's recent analysis that ranks AI applications to reduce dosage errors and support administrative workflow as having a high potential for producing tangible benefits during the next few years. The remainder of this article will focus on real applications of AI to prevent medication errors and predict risks to help avoid adverse events.
Preventing medication errors
Rules-based decision support found today in electronic prescribing systems is generally effective in triggering alerts based on a limited set of discrete factors evident at one point in time (e.g., drug-drug or drug-allergy interactions, therapeutic duplication, etc.). Clinically significant errors are missed because of the inherent limitations of these systems in detecting complex, time-dependent or uncommon medication errors. A new generation of AI-based medication decision support to close these gaps is emerging.
A team at the Brigham and Women’s Hospital evaluated a medication error detection system that uses a probabilistic machine-learning model to identify prescriptions that are outliers based on populations of patients with similar characteristics. This system detects potential medication errors due to changes in lab results over time, failure to monitor/change dosage as therapy progresses, and the prescribing of medicines to patients with clinical characteristics not normally evident for the ordered drug. Less false alerts should occur because the algorithms are continuously self-adjusting based on clinicians’ responses to the recommendations and retroactive analysis of error patterns.
Computer scientists at Stanford University have developed a machine-based learning application that infers potential side effects of drug combinations based on how each drug targets specific proteins in the body. With the myriad combinations of drugs used in medication therapy, accurately detecting potential side effects is beyond the capabilities of most decision support systems. For the 23% of Americans who take three or more prescription drugs, treatment of any polypharmacy side effects often happens after the fact. With Stanford’s advance, clinicians can more proactively manage the risks of polypharmacy at the point of prescribing.
Common to both of these innovative uses of AI is the targeting of multi-faceted medication problems that can benefit from algorithmic, machine-learning based models drawing on large data sets of patient populations. These and similar applications of AI to detect medication errors are a preview of next-generation drug utilization review, which will hopefully reduce the false positives and gaps in medication errors detection of current systems.
Preventing Drug Overdoses
Another way that AI is contributing to patient safety is using medication history to predict the potential for opioid overdoses before they happen, augmenting clinicians’ efforts to detect this life-threatening risk. Using patients’ medical and prescription history from various sources (e.g., EHR, claims, PDMP systems), algorithms are calculating “overdose risk scores”and predicting a patient’s risk of unintentionally overdosing from a prescribed opioid. For example, clinical notes in the EHR may contain critical past observations (e.g., change in patient’s ability to recall past treatments) that when codified using NLP enhances the power to detect possible overdose risks. A logical next step for this use of AI is also to recommend interventions based on past outcomes of patients with the same or similar set of risk factors for prescription opioid overdose.
The use of AI to determine the risk of opioid overdose and recommend interventions exemplifies where machine learning and NLP are well-suited to create value in medication management by augmenting clinical decision-making. The numerous risk factors contributing to prescribed drug overdoses plus the multiple types and sources of a patient’s health history needed to detect true risk makes it very challenging for a clinician to assess the risk of overdose in a timely fashion, let alone based on an assessment during a patient’s visit. Dr. Elizabeth Marshall, director of clinical analytics at an AI technology firm nicely summarizes the realistic role of AI, “when it comes to technology and NLP and artificial intelligence, I don’t think we should remove the human factor, but it can enhance our capabilities as humans.”
Barriers and Challenges
HIMSS Analytics recently reported two of the top barriers to adopting AI as “opportunities hard to understand” and “unproven business case.” The AI applications to augment clinical decisions discussed here are relatively straightforward, help solve persistent problems in medication management, and have measurable benefits. You are probably thinking at this point about other barriers, particularly the liquidity and quality of data necessary to train and optimize results with these AI applications. Stay tuned for future posts to POCP’s blog as I discuss the data challenges and efforts to overcome them. I’ll also explore the use of AI to streamline drug prior authorization and to improve medication adherence.
In the meantime, feel free to e-mail me at michael.solomon@pocp.com with your observations and/or questions.